Threat Hunting Frameworks: Strategies and Solutions
Published on
Published on
Published on
Sep 24, 2024
Sep 24, 2024
Sep 24, 2024
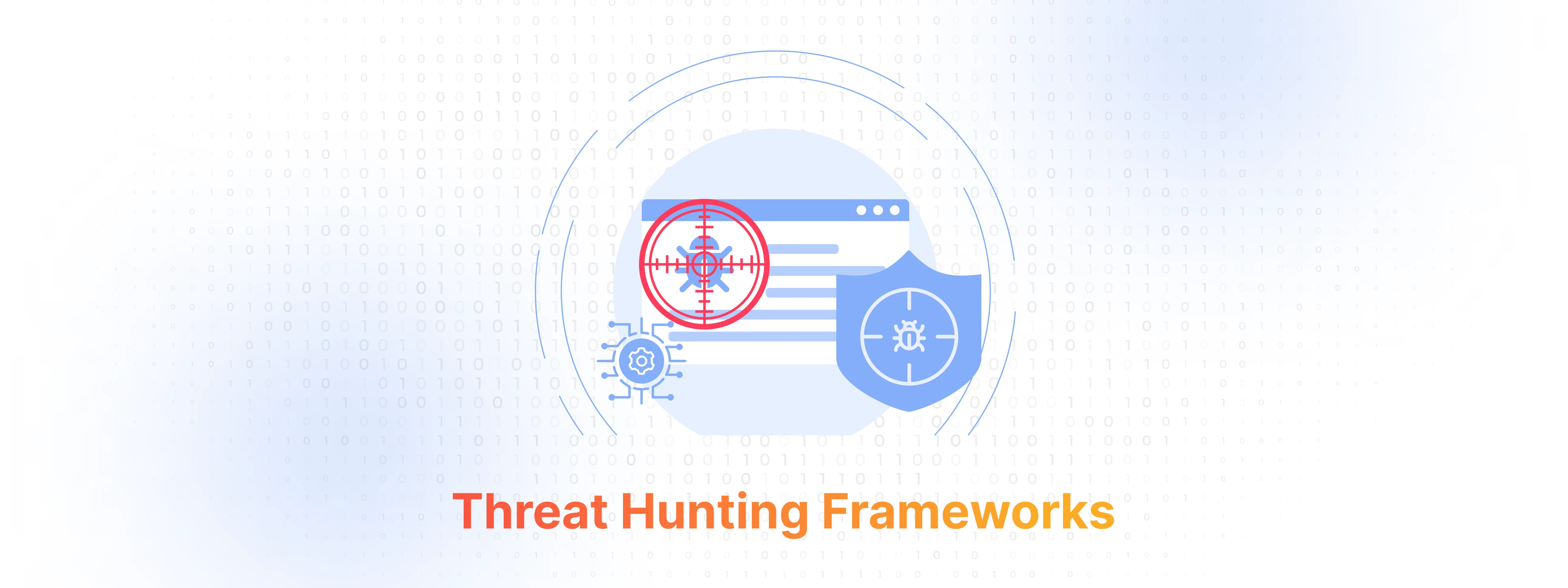
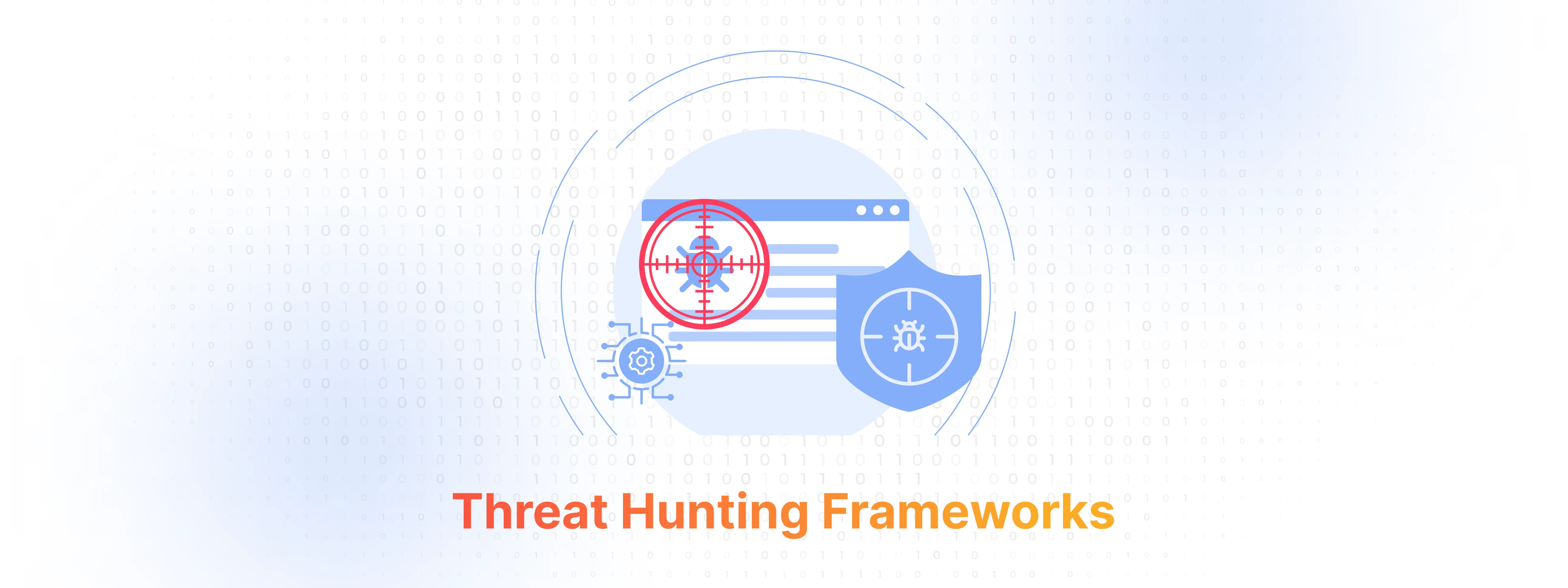
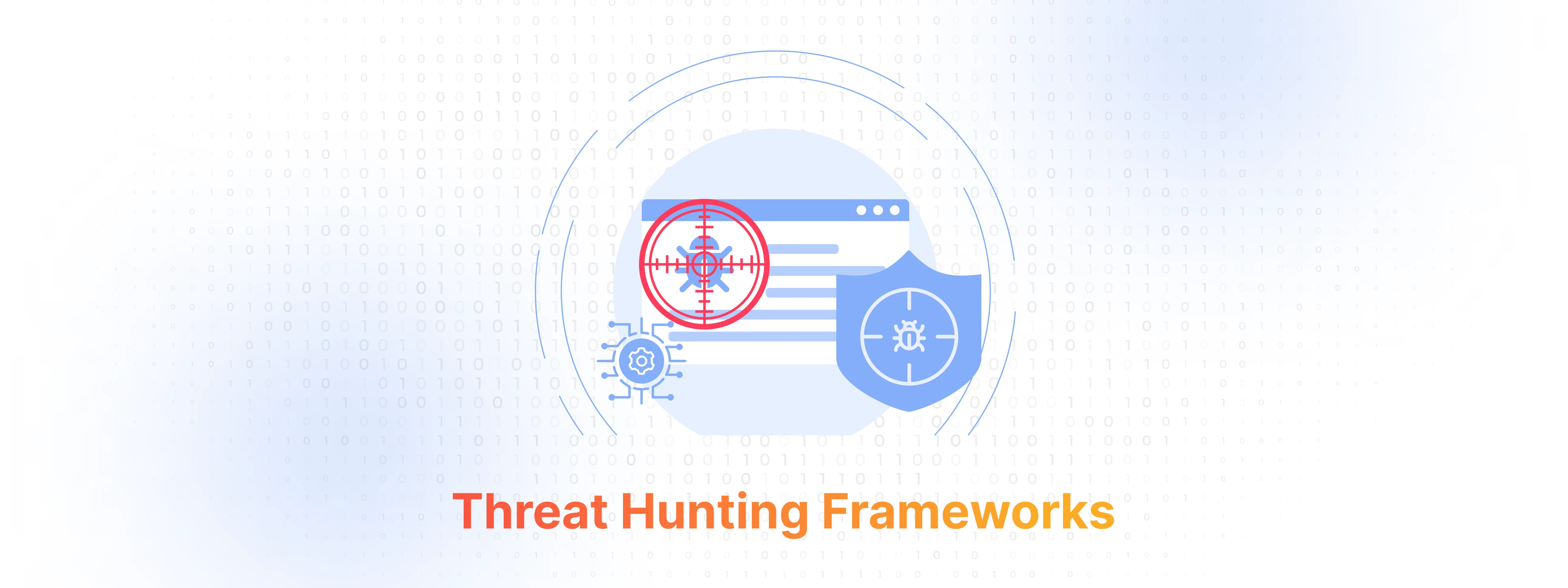



eBook
Modern Threat Hunting
Modern Threat Hunting
10 Practical Steps to Outsmart Adversaries
10 Practical Steps to Outsmart Adversaries
A Hands-On Guide Using Hunt.io’s Threat Intelligence Platform
Get the Free eBook
Get the Free eBook
Threat hunting frameworks help security teams find and respond to threats methodically and efficiently, guiding them to detect advanced threats that automated tools often miss. However, many organizations struggle with implementing these frameworks, as 50% report a lack of skilled staff as the top barrier to success.
To bridge this gap, it's crucial to understand how to build a framework that not only addresses these challenges but also strengthens your team's ability to hunt threats effectively. In this article, we'll explore the key components, strategies, and solutions for doing just that.
Key Facts
A structured threat hunting framework helps security teams to find relevant threats, model adversary tactics, and optimize incident response.
Key components of threat hunting are hypothesis-driven analysis, baseline behavior assessment, data collection, and integration of advanced technologies like machine learning and MITRE ATT&CK framework.
Continuous improvement and adaptation of threat hunting is key to defending against evolving threats, learning from past hunts, and staying up to date on emerging risks.
What is a Threat Hunting Framework?
A threat hunting framework is a set of repeatable processes to improve cyber threat hunting. These frameworks guide security teams to focus on relevant threats and add to the overall security infrastructure by providing insights and feedback to existing systems. By finding meaningful activity patterns and advanced threats that bypass automated security controls, threat hunting frameworks improve an organization's security.
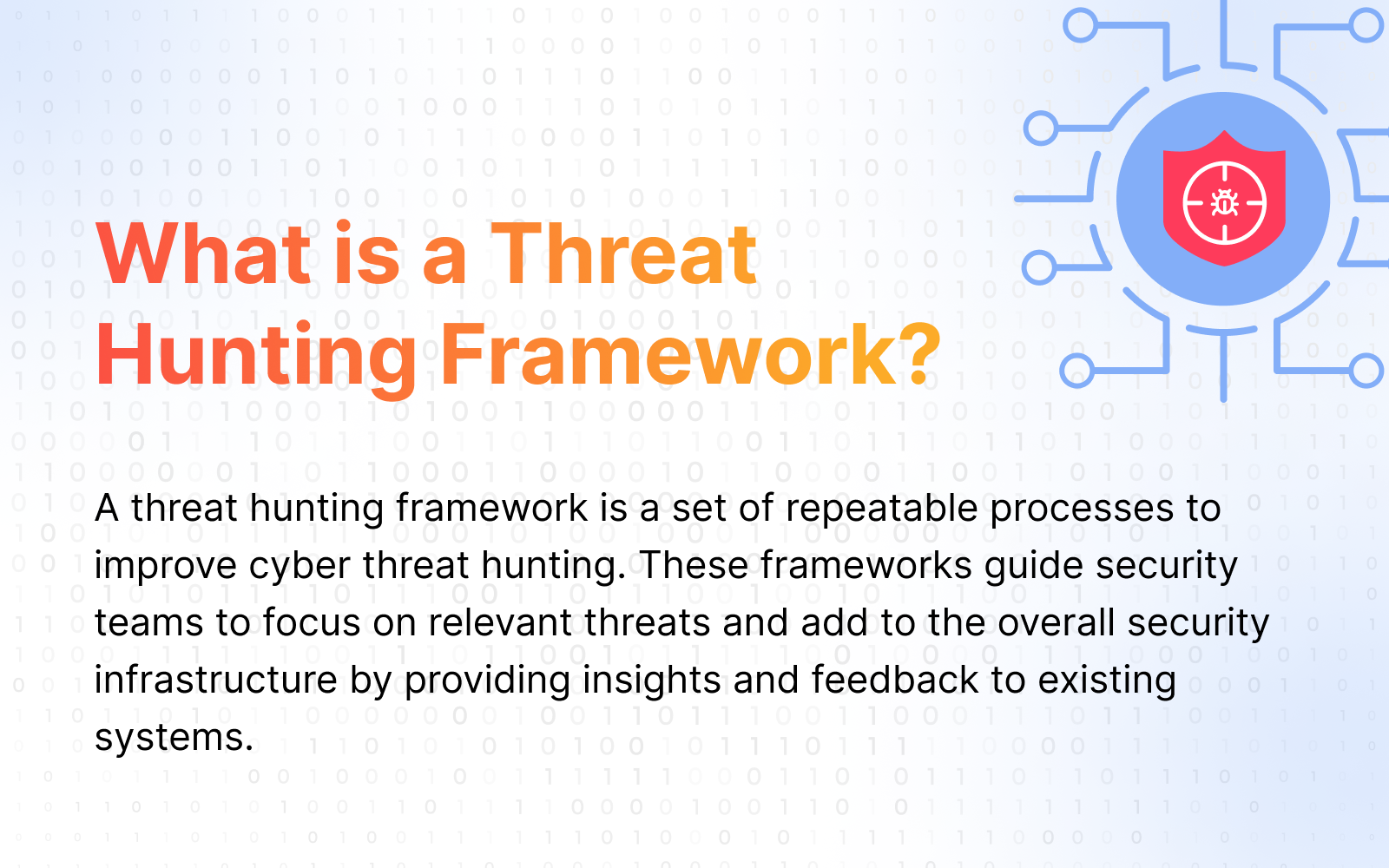
At its core, a well-structured threat hunting framework enables goal setting, measurement of outcomes, and presentation of findings. It allows security teams to:
Baseline normal behavior
Predict potential threats
Model adversary tactics
Find vulnerabilities
Optimize incident response
Threat hunting assumes a structured and systematic approach, a thorough investigation of every potential unstructured threat hunting.
The Role of Hypothesis-Driven Threat Hunting for Building Frameworks
Hypothesis-driven threat hunting is about forming a hypothesis about potential threats and using data and analysis to prove or disprove it. This is the foundation of the hypothesis-driven hunting process where situational hypotheses are built based on internal risk assessments or trends and vulnerabilities analysis.
For example, a threat hunter might hypothesize that a certain type of attack will happen based on recent threat intelligence or observed adversary tactics. In the case of SparkRAT, the Hunt.io research team applied this approach to track down malware infrastructure by correlating command and control (C2) servers and analyzing communication patterns. This methodical investigation led to identifying hidden infrastructure and validating the effectiveness of hypothesis-driven hunting.
Hypothesis-driven hunting succeeds by using relevant data sources and getting to data quickly. Investigations start from sources like threat intelligence, past incidents, and red teaming activities.
Validating a hypothesis requires multiple forms of evidence, using Indicators of Attack (IoAs) and Tactics, Techniques, and Procedures (TTP) of the attackers. This is one of the most popular types of threat hunting and ensures threat hunters can find vulnerabilities and mitigate potential threats before they escalate.
Baseline Behavior Analysis
Knowing normal behavior in the network is key to differentiating between benign anomalies and threats. Understanding adversary tradecraft and normal behavior allows threat hunters to use behavioral analysis to find irregularities that indicate malicious activity.
This allows security teams to detect and respond to potential threats faster, any deviation from the baseline behavior will be investigated.
Model-Assisted Threat Hunts
Combining machine learning with traditional methods improves threat-hunting detection. Machine learning can process huge amounts of data quickly and find unusual patterns that indicate malicious activity. Combining machine learning with human expertise makes the threat hunting process more efficient and effective.
This allows security teams to hunt and mitigate advanced persistent threats and threat actor activity before they can cause harm.
What is an Effective Threat Hunting Framework?
Threat hunting needs a clear structure, defined roles, standard operating procedures (SOPs) and a collaborative culture.
Key components of a threat hunting framework are:
Intelligence-driven hypotheses
Supporting data
Adversary model-based correlation
Field tested scenarios
Automation
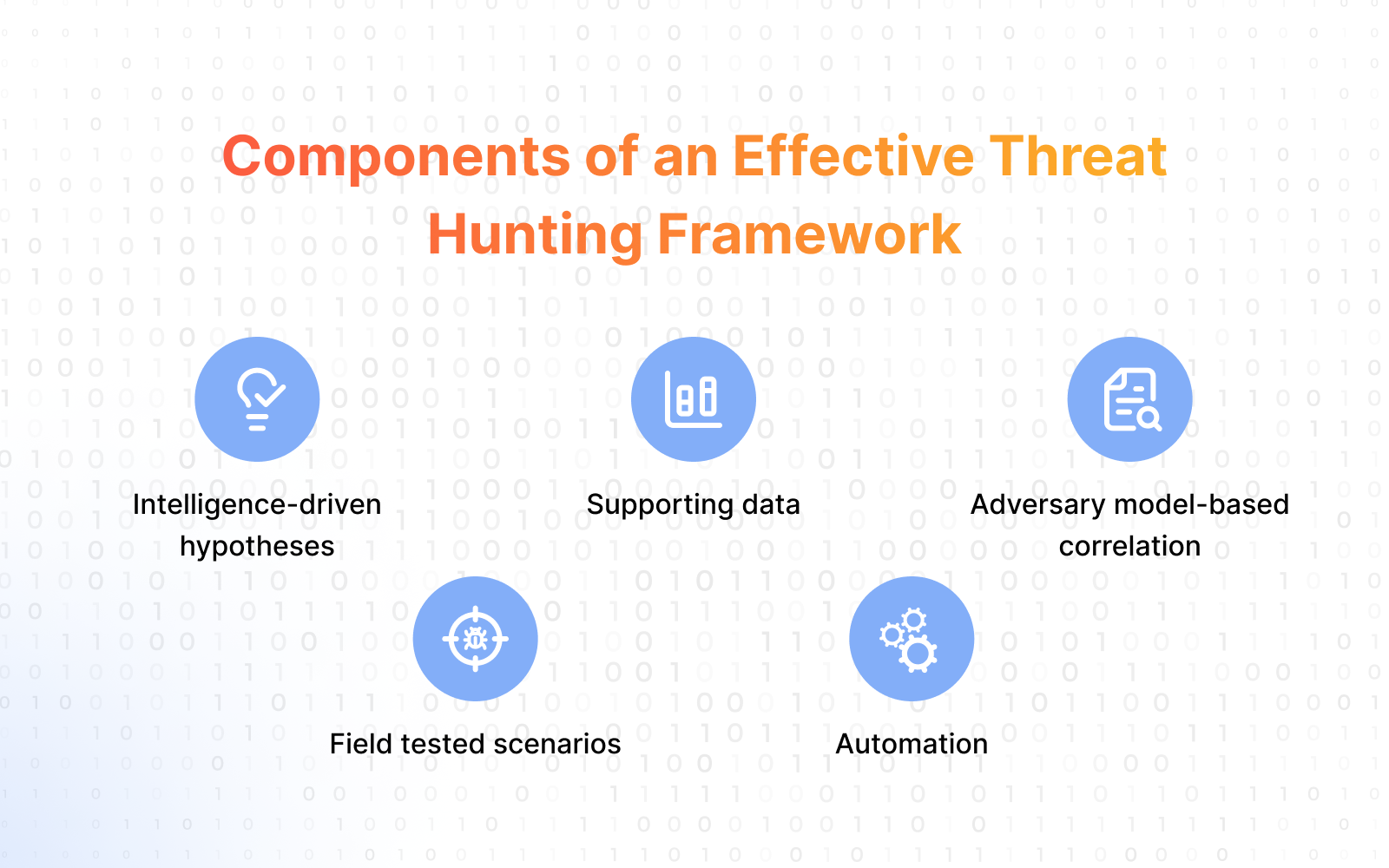
These components work together to create a complete and efficient threat hunting process, so security teams can defend against threat actors with various techniques and resources as needed.
Automation improves threat hunting efficiency by getting too good and bad activity quickly. But a structured threat hunting approach can be too rigid and miss new threats.
Information sharing and learning within the team are key to a threat hunting framework.
Data Collection and Management
Collecting relevant and actionable data from multiple sources like network logs, endpoint data, and threat intelligence reports is crucial for threat hunting. Getting to data quickly is key to fast investigation and decision-making so threat hunters can validate hypotheses and find potential threats. Security analytics tools are part of this process, using algorithms and analytics to find vulnerabilities in the IT systems.
Data sources for hypothesis validation can include alerts, flags, open-source data, forums, and hearsay. Good data gathering and management support investigations and the overall security of the organization. A structured data collection and management process is key to threat hunting.
Correlation with Threat Intelligence
Reviewing and staying up to date with the latest threat intelligence feeds is important for threat hunting. Threat intelligence sharing helps organizations know about new vulnerabilities and cyber trends. Data for threat hunting hypotheses can be found in threat intelligence feeds, forums, social media, and RSS feeds.
The MITRE ATT&CK framework informs threat hunting and predicts adversary behavior so hunters can correlate TTPs.
Scenario Creation and Testing
Creating scenarios in threat hunting validates or invalidates threats based on hypotheses. The investigation phase validates hypotheses. It also determines the scope of the threat.
Advanced analytical skills are required in this phase to get a deep and broad view of the threat. Hunt.io's investigation of the Oyster backdoor is a great example of this in action. By analyzing TLS certificates and HTML body hashes, we were able to uncover previously unknown domains and C2 servers tied to the backdoor's malicious infrastructure. This illustrates how scenario testing, combined with intelligence gathering, can expose sophisticated attack frameworks
Scenarios often use multiple theories to get a full picture.
Frameworks for Advanced Threat Hunting
Advanced threat hunting uses advanced tools and methods to improve detection and response. Common tools are Endpoint Detection and Response (EDR) solutions and Security Information and Event Management (SIEM) systems. Network traffic analysis tools are also used. These tools provide real-time monitoring and threat intelligence integration. They also have automated responses which makes threat hunting much more efficient.
Threat hunting is a mix of human creativity and automated analysis tools. Using advanced tools and threat hunting techniques helps security teams stay ahead of cyber threats and better protect their organizations.
Machine Learning and AI
Machine learning in threat hunting can analyze massive amounts of data in seconds to find anomalies. Model-Assisted Threat Hunts combines hypothesis-driven and baseline approaches with automation so data analysis is efficient. AI can automate parts of the threat hunting workflow to make the process more efficient.
Despite AI, human skills are still key in threat hunting. AI is a tool, not a replacement and human intuition adds a lot of value to the process. Using contextual information and human expertise makes the threat hunting process more effective and broader.
Using MITRE ATT&CK Framework
The MITRE ATT&CK framework is used to structure threat hunting and predict adversary behavior. Structured Threat Hunting uses this framework to refine detection and avoid biases. Using adversarial models helps to focus the data collection on specific threats and predict attacker behavior.
Intelligence for Threat Hunting Frameworks
A threat hunting framework means intelligence-led hypotheses, relevant data, adversarial correlations, practical scenarios, and automation. Managed detection and response services provide outsourced threat hunting support to the organization.
Things to consider when implementing is to tailor the process to the organization's risk profile and security landscape. Having a framework allows the organization to defend against cyber threats and improve overall security.
Security Data Lake
A centralized security data repository or security data lake allows the organization to operationalize and manage security data. Combining security and business data in a security data lake makes threat assessment and long-term storage of large amounts of historical security data possible.
This gives a full view of security information.
Automating Low-Level Tasks
Automating mundane threat hunting tasks allows human experts to focus on more complex investigations. By reducing the time spent on routine tasks, automation allows analysts to focus on more critical issues and makes threat hunting more efficient.
Continuous Improvement in Threat Hunting
Continuous improvement in threat hunting is key to staying ahead of the evolving cyber threats and improving overall security posture. Proactive threat hunting reduces the average time an attacker is undetected which can be up to 280 days. Feedback from previous threat hunting activities makes the next hunts more effective.
Threat hunting practices need to be reviewed regularly to stay ahead of cyber threats. By improving their threat hunting strategy organizations can have a strong security posture.
Lessons from Previous Hunts
Reviewing previous threat hunting activities helps to refine the techniques and improve future threat detection and response. Lessons from previous hunts give valuable insights and show patterns and anomalies that can be used to inform future strategies. Reviewing previous hunts helps to continuous improvement.
Staying up to date with Emerging Threats
Continuous education on new threats and vulnerabilities is required for cyber threat hunters to be able to predict and respond to emerging cyber risks. Engaging with industry communities and resources keeps the threat hunters informed of the latest threats and helps them to protect their organization.
Related Questions
What are the components of a threat hunting framework?
A threat hunting framework has intelligence-led hypotheses, supporting data, adversarial model-based correlation, practical scenarios, and automation. All these components make a full and efficient threat hunting process.
How does hypothesis-driven threat hunting work?
Hypothesis-driven threat hunting is an approach where you formulate a hypothesis about the threats and then use data and analysis to prove or disprove that hypothesis. This uses Indicators of Attack (IoAs) along with the Tactics, Techniques, and Procedures (TTP) used by the attackers.
What role does machine learning play in threat hunting?
Machine learning helps in threat hunting by automating big data analysis to find anomalies and thus improve detection. However human analyst expertise is still required as AI is a tool, not a replacement.
Why is continuous improvement important in threat hunting?
Continuous improvement is important in threat hunting as it allows the organization to stay up to date with emerging threats and improve its security posture. By learning from previous hunts and staying up to date with emerging risks a better and more agile threat hunting strategy can be had.
Wrapping up
In summary, a threat hunting framework is a must-have for any organization to have a strong security posture. By using intelligence-led hypotheses, relevant data, adversarial correlations, practical scenarios, and automation the organization can have a full and efficient threat-hunting process---continuous improvement, learning from previous hunts, and staying up to date with emerging threats.
Threat hunting is a journey and the cyber threat landscape is always changing. By being proactive and using advanced techniques and tools the security teams can stay ahead of the threats and protect their organization better. The key to successful threat hunting is the combination of human creativity, advanced technology, and continuous learning.
There needs to be more than threat Hunting Frameworks. Hunt.io's Threat Hunting Platform goes beyond manual processes, offering real-time insights, automation, and advanced threat intelligence. Empower your team to hunt smarter and stay ahead of evolving threats. Book a demo today.
Threat hunting frameworks help security teams find and respond to threats methodically and efficiently, guiding them to detect advanced threats that automated tools often miss. However, many organizations struggle with implementing these frameworks, as 50% report a lack of skilled staff as the top barrier to success.
To bridge this gap, it's crucial to understand how to build a framework that not only addresses these challenges but also strengthens your team's ability to hunt threats effectively. In this article, we'll explore the key components, strategies, and solutions for doing just that.
Key Facts
A structured threat hunting framework helps security teams to find relevant threats, model adversary tactics, and optimize incident response.
Key components of threat hunting are hypothesis-driven analysis, baseline behavior assessment, data collection, and integration of advanced technologies like machine learning and MITRE ATT&CK framework.
Continuous improvement and adaptation of threat hunting is key to defending against evolving threats, learning from past hunts, and staying up to date on emerging risks.
What is a Threat Hunting Framework?
A threat hunting framework is a set of repeatable processes to improve cyber threat hunting. These frameworks guide security teams to focus on relevant threats and add to the overall security infrastructure by providing insights and feedback to existing systems. By finding meaningful activity patterns and advanced threats that bypass automated security controls, threat hunting frameworks improve an organization's security.
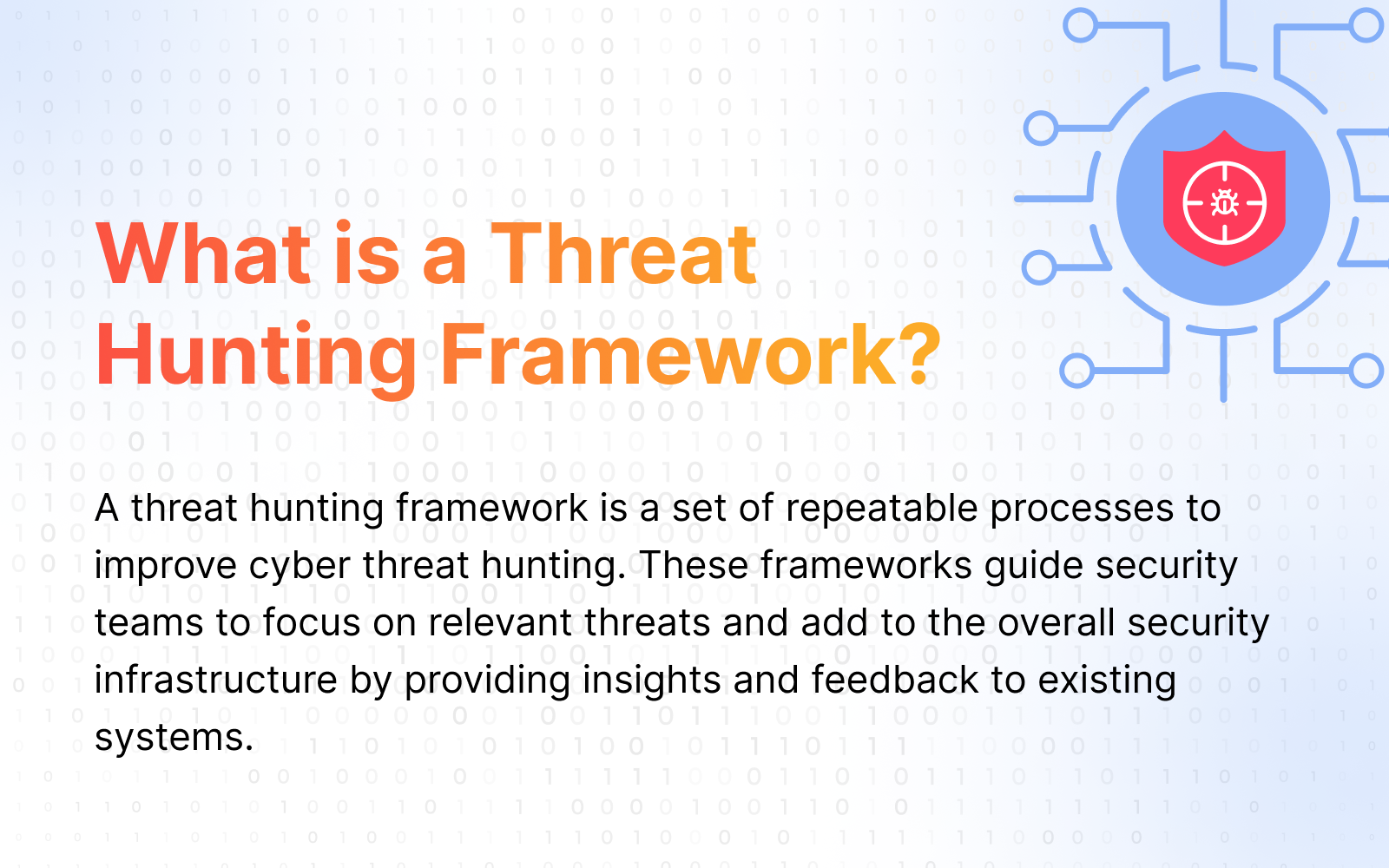
At its core, a well-structured threat hunting framework enables goal setting, measurement of outcomes, and presentation of findings. It allows security teams to:
Baseline normal behavior
Predict potential threats
Model adversary tactics
Find vulnerabilities
Optimize incident response
Threat hunting assumes a structured and systematic approach, a thorough investigation of every potential unstructured threat hunting.
The Role of Hypothesis-Driven Threat Hunting for Building Frameworks
Hypothesis-driven threat hunting is about forming a hypothesis about potential threats and using data and analysis to prove or disprove it. This is the foundation of the hypothesis-driven hunting process where situational hypotheses are built based on internal risk assessments or trends and vulnerabilities analysis.
For example, a threat hunter might hypothesize that a certain type of attack will happen based on recent threat intelligence or observed adversary tactics. In the case of SparkRAT, the Hunt.io research team applied this approach to track down malware infrastructure by correlating command and control (C2) servers and analyzing communication patterns. This methodical investigation led to identifying hidden infrastructure and validating the effectiveness of hypothesis-driven hunting.
Hypothesis-driven hunting succeeds by using relevant data sources and getting to data quickly. Investigations start from sources like threat intelligence, past incidents, and red teaming activities.
Validating a hypothesis requires multiple forms of evidence, using Indicators of Attack (IoAs) and Tactics, Techniques, and Procedures (TTP) of the attackers. This is one of the most popular types of threat hunting and ensures threat hunters can find vulnerabilities and mitigate potential threats before they escalate.
Baseline Behavior Analysis
Knowing normal behavior in the network is key to differentiating between benign anomalies and threats. Understanding adversary tradecraft and normal behavior allows threat hunters to use behavioral analysis to find irregularities that indicate malicious activity.
This allows security teams to detect and respond to potential threats faster, any deviation from the baseline behavior will be investigated.
Model-Assisted Threat Hunts
Combining machine learning with traditional methods improves threat-hunting detection. Machine learning can process huge amounts of data quickly and find unusual patterns that indicate malicious activity. Combining machine learning with human expertise makes the threat hunting process more efficient and effective.
This allows security teams to hunt and mitigate advanced persistent threats and threat actor activity before they can cause harm.
What is an Effective Threat Hunting Framework?
Threat hunting needs a clear structure, defined roles, standard operating procedures (SOPs) and a collaborative culture.
Key components of a threat hunting framework are:
Intelligence-driven hypotheses
Supporting data
Adversary model-based correlation
Field tested scenarios
Automation
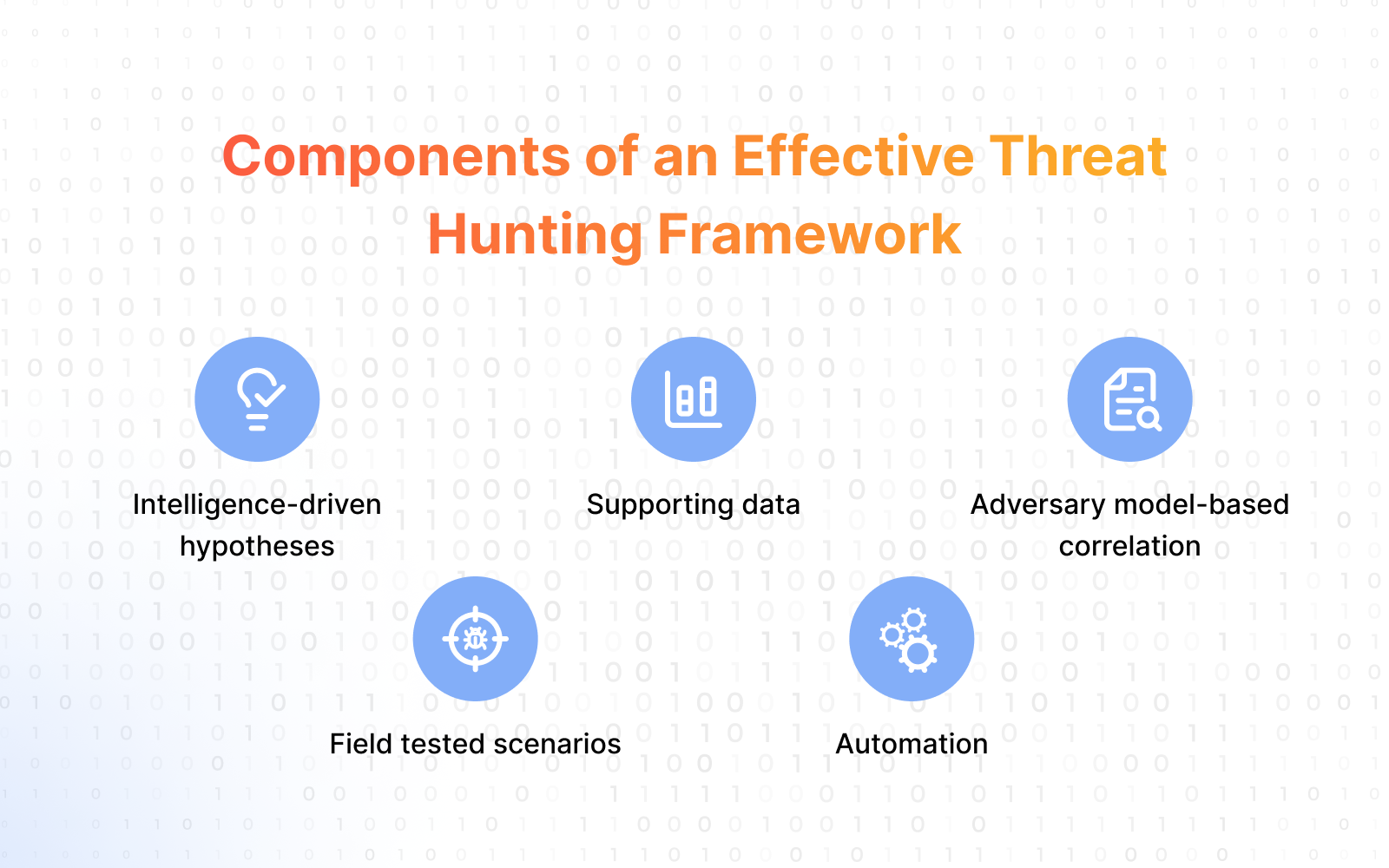
These components work together to create a complete and efficient threat hunting process, so security teams can defend against threat actors with various techniques and resources as needed.
Automation improves threat hunting efficiency by getting too good and bad activity quickly. But a structured threat hunting approach can be too rigid and miss new threats.
Information sharing and learning within the team are key to a threat hunting framework.
Data Collection and Management
Collecting relevant and actionable data from multiple sources like network logs, endpoint data, and threat intelligence reports is crucial for threat hunting. Getting to data quickly is key to fast investigation and decision-making so threat hunters can validate hypotheses and find potential threats. Security analytics tools are part of this process, using algorithms and analytics to find vulnerabilities in the IT systems.
Data sources for hypothesis validation can include alerts, flags, open-source data, forums, and hearsay. Good data gathering and management support investigations and the overall security of the organization. A structured data collection and management process is key to threat hunting.
Correlation with Threat Intelligence
Reviewing and staying up to date with the latest threat intelligence feeds is important for threat hunting. Threat intelligence sharing helps organizations know about new vulnerabilities and cyber trends. Data for threat hunting hypotheses can be found in threat intelligence feeds, forums, social media, and RSS feeds.
The MITRE ATT&CK framework informs threat hunting and predicts adversary behavior so hunters can correlate TTPs.
Scenario Creation and Testing
Creating scenarios in threat hunting validates or invalidates threats based on hypotheses. The investigation phase validates hypotheses. It also determines the scope of the threat.
Advanced analytical skills are required in this phase to get a deep and broad view of the threat. Hunt.io's investigation of the Oyster backdoor is a great example of this in action. By analyzing TLS certificates and HTML body hashes, we were able to uncover previously unknown domains and C2 servers tied to the backdoor's malicious infrastructure. This illustrates how scenario testing, combined with intelligence gathering, can expose sophisticated attack frameworks
Scenarios often use multiple theories to get a full picture.
Frameworks for Advanced Threat Hunting
Advanced threat hunting uses advanced tools and methods to improve detection and response. Common tools are Endpoint Detection and Response (EDR) solutions and Security Information and Event Management (SIEM) systems. Network traffic analysis tools are also used. These tools provide real-time monitoring and threat intelligence integration. They also have automated responses which makes threat hunting much more efficient.
Threat hunting is a mix of human creativity and automated analysis tools. Using advanced tools and threat hunting techniques helps security teams stay ahead of cyber threats and better protect their organizations.
Machine Learning and AI
Machine learning in threat hunting can analyze massive amounts of data in seconds to find anomalies. Model-Assisted Threat Hunts combines hypothesis-driven and baseline approaches with automation so data analysis is efficient. AI can automate parts of the threat hunting workflow to make the process more efficient.
Despite AI, human skills are still key in threat hunting. AI is a tool, not a replacement and human intuition adds a lot of value to the process. Using contextual information and human expertise makes the threat hunting process more effective and broader.
Using MITRE ATT&CK Framework
The MITRE ATT&CK framework is used to structure threat hunting and predict adversary behavior. Structured Threat Hunting uses this framework to refine detection and avoid biases. Using adversarial models helps to focus the data collection on specific threats and predict attacker behavior.
Intelligence for Threat Hunting Frameworks
A threat hunting framework means intelligence-led hypotheses, relevant data, adversarial correlations, practical scenarios, and automation. Managed detection and response services provide outsourced threat hunting support to the organization.
Things to consider when implementing is to tailor the process to the organization's risk profile and security landscape. Having a framework allows the organization to defend against cyber threats and improve overall security.
Security Data Lake
A centralized security data repository or security data lake allows the organization to operationalize and manage security data. Combining security and business data in a security data lake makes threat assessment and long-term storage of large amounts of historical security data possible.
This gives a full view of security information.
Automating Low-Level Tasks
Automating mundane threat hunting tasks allows human experts to focus on more complex investigations. By reducing the time spent on routine tasks, automation allows analysts to focus on more critical issues and makes threat hunting more efficient.
Continuous Improvement in Threat Hunting
Continuous improvement in threat hunting is key to staying ahead of the evolving cyber threats and improving overall security posture. Proactive threat hunting reduces the average time an attacker is undetected which can be up to 280 days. Feedback from previous threat hunting activities makes the next hunts more effective.
Threat hunting practices need to be reviewed regularly to stay ahead of cyber threats. By improving their threat hunting strategy organizations can have a strong security posture.
Lessons from Previous Hunts
Reviewing previous threat hunting activities helps to refine the techniques and improve future threat detection and response. Lessons from previous hunts give valuable insights and show patterns and anomalies that can be used to inform future strategies. Reviewing previous hunts helps to continuous improvement.
Staying up to date with Emerging Threats
Continuous education on new threats and vulnerabilities is required for cyber threat hunters to be able to predict and respond to emerging cyber risks. Engaging with industry communities and resources keeps the threat hunters informed of the latest threats and helps them to protect their organization.
Related Questions
What are the components of a threat hunting framework?
A threat hunting framework has intelligence-led hypotheses, supporting data, adversarial model-based correlation, practical scenarios, and automation. All these components make a full and efficient threat hunting process.
How does hypothesis-driven threat hunting work?
Hypothesis-driven threat hunting is an approach where you formulate a hypothesis about the threats and then use data and analysis to prove or disprove that hypothesis. This uses Indicators of Attack (IoAs) along with the Tactics, Techniques, and Procedures (TTP) used by the attackers.
What role does machine learning play in threat hunting?
Machine learning helps in threat hunting by automating big data analysis to find anomalies and thus improve detection. However human analyst expertise is still required as AI is a tool, not a replacement.
Why is continuous improvement important in threat hunting?
Continuous improvement is important in threat hunting as it allows the organization to stay up to date with emerging threats and improve its security posture. By learning from previous hunts and staying up to date with emerging risks a better and more agile threat hunting strategy can be had.
Wrapping up
In summary, a threat hunting framework is a must-have for any organization to have a strong security posture. By using intelligence-led hypotheses, relevant data, adversarial correlations, practical scenarios, and automation the organization can have a full and efficient threat-hunting process---continuous improvement, learning from previous hunts, and staying up to date with emerging threats.
Threat hunting is a journey and the cyber threat landscape is always changing. By being proactive and using advanced techniques and tools the security teams can stay ahead of the threats and protect their organization better. The key to successful threat hunting is the combination of human creativity, advanced technology, and continuous learning.
There needs to be more than threat Hunting Frameworks. Hunt.io's Threat Hunting Platform goes beyond manual processes, offering real-time insights, automation, and advanced threat intelligence. Empower your team to hunt smarter and stay ahead of evolving threats. Book a demo today.
Related Posts:
Get biweekly intelligence to hunt adversaries before they strike.
Products
Hunt Intelligence, Inc.
Get biweekly intelligence to hunt adversaries before they strike.
Products
Hunt Intelligence, Inc.
Get biweekly intelligence to hunt adversaries before they strike.
Products
Hunt Intelligence, Inc.